Language barriers can be overcome with artificial intelligence. The best software does not come from Google, but from Cologne.
Translating any digital text into your own language with just one click: this dream became everyday life.
In mid-January there was an uproar at Swiss Post. The reason: The company management had blocked access to online translators such as DeepL and Google Translate. When Post employees clicked on it, they were automatically redirected to the company’s Post Translate service. The employees protested violently. The decision was reversed.
What at first glance sounds like an anecdote about the sluggishness of state-owned companies shows something else: how much automatic translators have crept into the everyday lives of many employees.
They unwaveringly translate business correspondence and declarations of love. It has become normal to make foreign language websites and articles readable in your own language with one click. Zurich high school teacher Philippe Wampfler reports in one blog post even that students use the DeepL program to first translate their texts into English and then back into German because this corrects punctuation errors and improves the choice of words.
Curious translations on menus, such as “Tagliatelle with sponge” and “Lasagna in the oven”, which once cheered up dinner in beach restaurants, have become rare.
The revolution in automatic translation ran quietly and casually. It is an example of what artificial intelligence (AI) is already able to do today. Far from the dream of real, human-like intelligence, but good enough to threaten an entire profession.
How neural networks revolutionized translation
Rico Sennrich experienced the silent revolution up close. In 2013 he obtained his PhD in the field of statistical translation. Three years later, the branch of research was obsolete. Artificial neural networks had supplanted him.
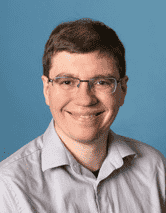
Sennrich became interested in the new methods early enough. He’s one of those guys who perfected them and helped them break through. Today he researches and teaches as a professor for computational linguistics at the University of Zurich.
In terms of the basic idea, automatic translators of the old, statistical variant and those of the new AI variant are similar, he explains. Because both use vast amounts of already translated sentence pairs, from websites, parliamentary speeches, film subtitles. And both use statistics to suggest the appropriate translation for a sentence or phrase.
But they do it very differently. The statistical model splits the sentences into word groups of two or three components, finds the most likely translation for these and puts everything back together. That’s why automatic translators were still spitting out things like, “Download error: no creek found,” around 2014.
“Download error. No stream found.” If the translation falls through, the stream just becomes a creek… pic.twitter.com/xM1Qy12Blb
— Oliver Straub (@OliverStraub) June 17, 2014
The statistical programs “saw” that the English word “stream” is used here in connection with “download”, so the word “Bach” is out of place.
This no longer happens with neural networks. In their probability calculation, they include the entire sentence, and in some cases the entire paragraph, that comes before the word they are looking for. The most plausible translation is therefore calculated depending on many more factors. As a result, neural networks suggest the right word much more often.
Science didn’t just come up with the idea that more context could deliver better translations in 2014. Rico Sennrich says: “Of course, some people suspected that neural networks could work here.” Back then, computers were still too slow for that. “Just trying out a test model would have taken a whole year,” says Sennrich. Today far more complex models are calculated in a few days.
The German startup that beats Google
In 2017, the Cologne translation service DeepL went online. And soon did headlines, because it delivered better results in blind tests than its competitors from Google and Microsoft. What’s behind it?
Basically, translators using neural networks consist of three components: The first part reads the sentence and creates a series of numbers, called a vector, for each word. Their meaning can hardly be interpreted by a human being. Only the second part of the model can do that. This converts the blocks of numbers back into possible word combinations, this time in the target language. In a final step, an algorithm checks which of the word combinations form the most plausible sentence. This appears in the output field.
To this end, the company operates several large data centers in Iceland, Finland and Sweden. According to managing director Jaroslaw Kutylowski, hardware, energy and other operating costs make up a substantial part of the effort.
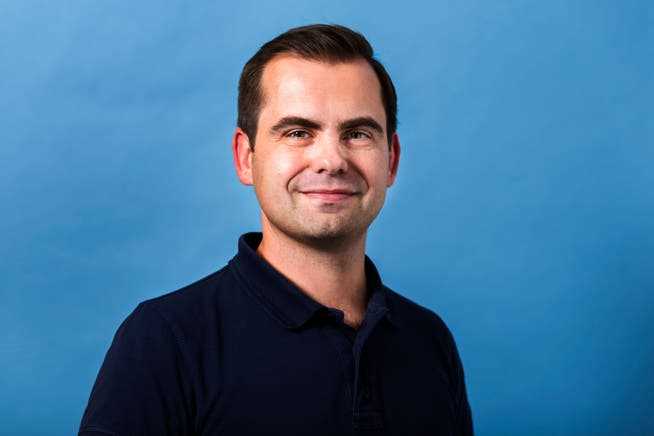
Yaroslav Kutylowski, Managing Director of DeepL.
About half of the computing capacity is needed for translating in action. The other half is used for research and development. The training of the artificial neural networks is particularly energy-intensive. In this it is determined with which calculation steps the number vectors, which were mentioned before, are to be calculated.
The advantage of good data
In order to train a neural network, it is presented with sentences and their translations. The model adjusts its adjusting screws according to the principle of trying out and refining. It will be rewarded if the translation it spits out is accurate, and will remember its mistake if it’s bad. In this way, the model gradually arrives at values that are suitable for translation. Even if there are new sentences that never appeared in the training data. At least that’s how it works at best.
And this is exactly where DeepL had an advantage. Because long before neural translators were developed under this name, the parent company was already successfully operating a kind of online dictionary called Linguee. Unlike a classic dictionary, however, Linguee doesn’t just put a couple of translations for each word online. Instead, the company searches the network for suitable translations, which are displayed in the context, for each request. As with the Google search, the core is the algorithm, which sorts the results according to relevance and quality.
For this service, the startup had to research algorithms that distinguish good translations from bad ones. It employed professional translators and engineers from the beginning to make this selection better and better. Their knowledge and the collected data were good starting points in the race for the best neural translator. Because even more than with statistical translation, neural networks require very high data quality – even a small proportion of meaningless translations can confuse a neural network during training.
Today automatic translation is so good that most translation agencies use it for their work. Humans are still needed to check what the algorithm has produced, to adapt technical language and to correct incorrect references.
Where the machine still fails
However, it is not yet possible to rely on neural networks, especially with long documents. Because they have some of the word environment “in view” but not the entire text. Another difficulty is recognizing and transmitting the tone of a text, also because languages are very different. English makes fewer distinctions between formal and informal expression than German, Japanese more. DeepL employs translators and developers to excel at such subtleties, says Kutylowski.
As intelligent as AI models that produce language may seem, in the end they are “stochastic parrots”, like American researchers have called: They don’t understand anything, but parrot, better or worse, depending on how you feed them.
But none of that was the problem that the Post Office had with foreign automatic translators. She was concerned with what happens to the information that the employees had translated. In fact, most free services store data permanently to improve their own offerings. However, Postfinance employees must meet the high security and data protection requirements imposed by banks. That’s why the decision was made to use a system from the French provider Systran, with servers in Geneva, writes the Post on request.
The postal workers, who have nothing to do with the banking sector, can now translate as they please again.